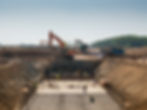
The construction industry constantly faces challenges in terms of efficiency, safety, and sustainability. To address these challenges, innovative solutions like machine learning algorithms have been developed, transforming the way projects are planned, built, and managed. In this article, we will explore how machine learning algorithms can improve construction and how SAALG Geomechanics' web platform, DAARWIN, is leading this transformation.
Project Planning Optimization:
One of the major advantages of machine learning algorithms is their ability to analyze large amounts of data and generate valuable insights for decision-making. In the context of construction, this means that machine learning algorithms can be used to optimize project planning. These algorithms can analyze historical data, site conditions, and other relevant factors to predict the duration, cost, and potential issues at each stage of the project. By anticipating these problems, construction teams can take preventive measures and minimize delays and additional costs.
Enhanced Safety and Risk Management:
Safety is a priority in the construction industry, and machine learning algorithms can play a crucial role in improving safety in projects. These algorithms can analyze safety data, such as past incidents, site conditions, and environmental factors, to identify patterns and potential risks. With this information, construction teams can implement appropriate safety measures and make informed decisions to minimize risks and protect the health and safety of workers and the general public.
Quality Control and Predictive Maintenance:
Another area where machine learning algorithms can make a difference is in quality control and predictive maintenance. These algorithms can analyze performance data from assets, such as machinery and equipment, to identify anomalies and predict potential failures. This allows maintenance to adopt a predictive approach rather than a reactive one. By anticipating possible issues, timely repairs and preventive maintenance can be performed, avoiding costly and prolonged project disruptions. Additionally, by utilizing machine learning algorithms to manage assets, companies can optimize equipment performance and extend their lifespan, resulting in better return on investment and increased operational efficiency.
DAARWIN: Empowering Construction Improvement
In the context of construction improvement through machine learning algorithms, it is important to mention the DAARWIN platform developed by SAALG Geomechanics. DAARWIN is a web platform that connects and centralizes geotechnical data throughout the project lifecycle. From initial exploration using historical information to correlation with real-time observation data during construction, DAARWIN analyzes these datasets to optimize construction procedures by reducing geotechnical uncertainties.
A notable feature of DAARWIN is its machine learning algorithm, which enhances the accuracy of predictive models in diagnosing oversized and unforeseen events. This technology minimizes risks and maximizes the sustainability and profitability of construction projects. By reducing overdesign, material consumption, and CO2 emissions, DAARWIN contributes to environmental preservation and climate change mitigation.
Furthermore, DAARWIN digitizes the entire project lifecycle, facilitating data-driven decision-making in a faster and easier manner. By integrating numerical models, construction progress, monitoring data, images, and historical information, professionals can analyze multiple design options and geotechnical parameter scenarios, from pessimistic to optimistic, to determine the most optimal design and influential geotechnical parameters.
geotechnical, software engineer, geotechnical engineering software, construction AI, civil engineering software